· 7 min read
Learning to Leverage AI with Prudent Proof-of-Concept Projects
You don't learn to ride your bike on the highway. Choose the right projects to safely build skills and confidence.
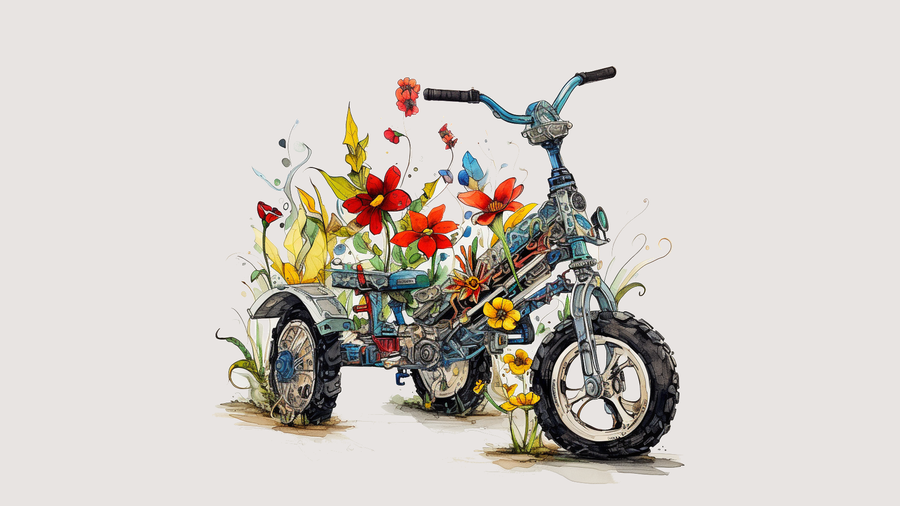
Adopt and Adapt
Few technologies have catapulted into the collective awareness like large language models (LLMs). In the months after OpenAI’s first release of ChatGPT, millions of users experienced the possibility of this technology first-hand. Within the legal industry, this possibility has been further evidenced through our research on the Bar Exam or pioneering products like CoCounsel.
However, as the initial AI-fueled adrenaline rush fades, law firms and legal departments are now sobering up to the reality that a more thoughtful approach will be required.
For some, the questions focus on whether and how to adopt these technologies in light of common ethical and compliance concerns. Once this threshold is passed, the focus is then typically on how to contract for AI models, train personnel, and integrate into real workflows.
For others, the questions are more fundamental: will their client expectations or competitive differentiation be impacted? If so, how should they adapt to this new reality?
Practice or Business of Law?
While the answers to these adopt-and-adapt questions will vary by firm and department, there is one common thread: all organizations need to develop a better understanding of this technology to build skills and confidence. And to do so, there is no better way than to actually use it in real-world projects.
However, this is where the rubber meets the road. From a top-down perspective, legal organizations typically divide their work into two categories: the practice of law and the business of law.
The practice of law is the core work of the organization. This is the work that clients pay for and the work that lawyers are theoretically trained to do. These activities are typically protected by privilege, and most likely to be regulated. More importantly, this is the work that is most likely to be scrutinized by clients and courts, as recent ChatGPT-related sanctions have demonstrated.
The business of law is, effectively, everything else. This is the work that supports the practice of law, like finance, marketing, knowledge management, and IT. Even though the names and organizational structures may vary across firms and departments, all organizations rely on these support functions to operate.
Much of the public discussion around AI in law has focused on the practice of law. Business of law activities, however, provide a much safer environment for learning and experimentation. While these projects still require compliance or information security review, in general, they are much less likely to create risks related to sensitive internal or client data. In many cases, these activities are also better suited to time-and-motion or quality assessments, which are critical to building confidence in the technology.
Pilot Projects for Legal AI
For these reasons and others, firms and departments are increasingly choosing to learn to leverage AI through business of law projects. We provide three exemplary projects below to illustrate the idea. While these real-world examples are drawn from our work with clients and Kelvin, they are likely to be relevant to nearly any legal organization.
1. Build an Internal Contract or Policy Database
Nearly every legal organization has a collection of contracts, policies, or other documents that are critical to their business. However, these documents are often scattered across different repositories, in different formats, and with different naming conventions. This makes it difficult to find and compare documents, and nearly impossible to analyze them at scale.
One of the most common and valuable projects we see involves the creation of a centralized knowledge management artifact for these documents. This typically involves a database, spreadsheet, or other system of record that contains the key information about each document or clause. LLMs can be used at many stages of this process, including:
- Identifying or classifying documents
- Identifying or classifying clauses within documents
- Extracting key terms from documents or clauses
- Comparing documents or clauses
In addition to allowing teams to develop experience and confidence, knowledge management projects like these also serve as a foundation for future AI projects. For example, organizations with their own contract or policy databases are much better positioned to train their own bespoke LLMs or implement their own contract playbooks or workflows.
2. Clean Historical Data
While many knowledge management projects focus on creating new artifacts, it is often even more valuable to focus on cleaning existing data. For example, almost all organizations have systems of record that contain historical information about matters, contracts, clients, or timekeeping. These systems are typically critical to the business, but also often contain significant amounts of missing or inaccurate data. As a result, both the business and legal teams are often unable to leverage data in these systems to make better decisions.
LLMs can be used to clean historical data in a variety of ways, including:
- Identifying data quality or consistency issues in matter or contract data
- Correcting or completing missing or inaccurate data from supporting narratives or documents
- Correcting or completing missing phase and task codes from timekeeping data
These projects almost always unlock value by improving the quality of existing tools or systems, such as fixing messy dashboards or decreasing cleanup efforts required for legal operations or data science teams. In addition, these projects also provide the opportunity for firms to leverage their historical data for the development of new, proprietary AI models.
3. Improve Billing Compliance and Efficiency
While knowledge management and IT projects often create indirect value, LLMs can also be used to create direct financial impact by improving billing compliance and efficiency. For example, many firms and legal departments have billing guidelines that are difficult to comply with, review, and enforce. Despite the importance of these guidelines, they are often inconsistently applied, resulting in significant write-offs, or require significant manual review, resulting in delays and inefficiencies.
LLMs can be used to improve billing compliance and efficiency in a variety of ways, including:
- Extracting key terms and rules from billing guideline documents
- Identifying potential billing guideline violations in timekeeping data
- Correcting or completing missing or inaccurate timekeeping data
- Correcting or completing missing phase and task codes from timekeeping data
Both law firms and legal departments can benefit from these efficiencies, as they allow for more accurate and timely billing, as well as more efficient review and enforcement of billing guidelines. Like in the projects above, this historical data can also serve as the basis for future, firm-specific AI models that can help them perform early case assessment or respond to RFP or pricing requests in more accurate, differentiated ways.
Getting on the Bike
While the adoption of AI in law might seem like an uphill journey at first, the best way to start is to simply get on the bike and start pedaling. By focusing on business of law projects, legal organizations can create an ideal environment for learning and experimentation, like training wheels on a quiet street.
In addition, these projects also provide the opportunity for organizations to develop their own training data, which can be used to build bespoke AI models in the future. As the competitive landscape and technical possibilities continue to evolve, the experience and assets created by these projects might turn out to be much more valuable than anyone expects.
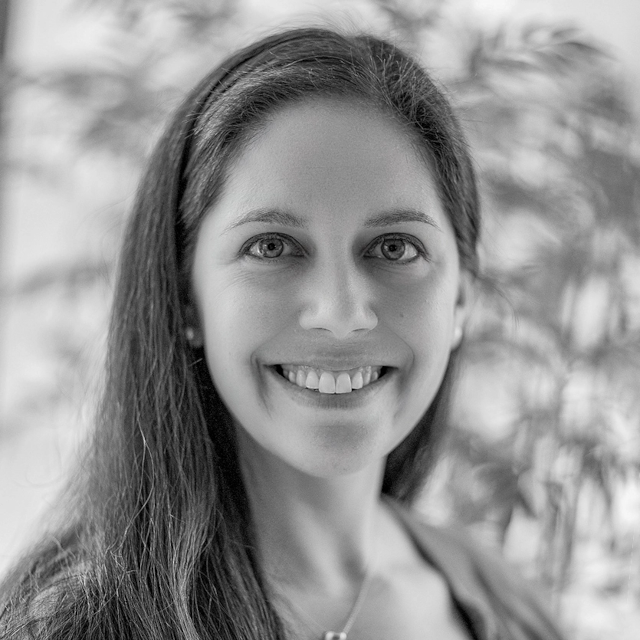
Jillian Bommarito, CPA, CIPP/US/E
Jillian is a Co-Founding Partner at 273 Ventures, where she helps ensure that Kelvin is developed and implemented in a way that is secure and compliant.
Jillian is a Certified Public Accountant and a Certified Information Privacy Professional with specializations in the United States and Europe. She has over 15 years of experience in the legal and accounting industries.
Would you like to learn more about risk management for AI-enabled legal tools? Send your questions to Jillian by email or LinkedIn.