· 13 min read
Common Requirements for Legal AI Solutions
Like snowflakes, every matter is unique - but the technology requirements behind them are not.
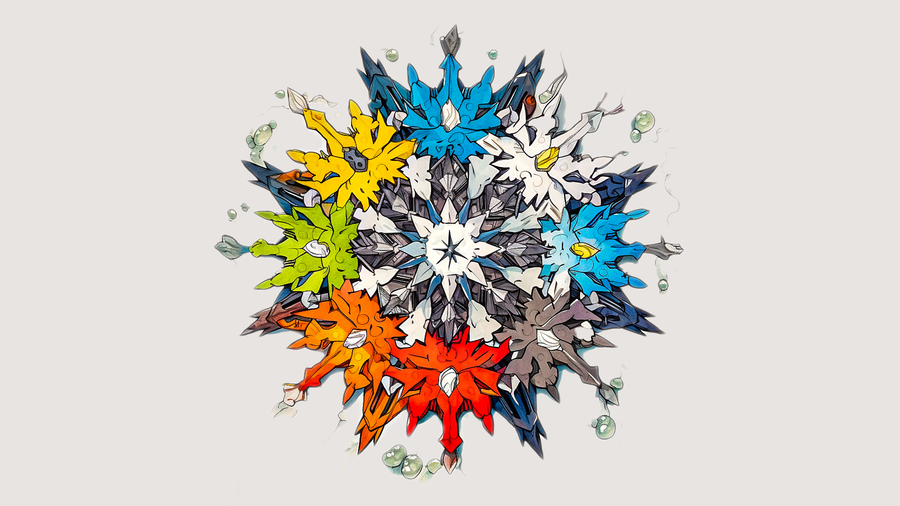
While you can always find some difference between the facts and circumstances of two cases, it’s much harder to find a truly unique risk or compliance requirement. In fact, most of the requirements that constrain how law firms and legal departments can procure and deploy legal AI solutions are frequently shared across matters and clients.
In this article, we’ll discuss some of the most common legal tech requirements that law firms and legal departments encounter and where they come from, especially as they relate to AI. By the time you’re done, you’ll have a better understanding of the pitfalls that commonly arise when evaluating or contracting for legal AI solutions.
The Five Sources of Requirements
Before we dive into the specific requirements, it’s important to understand where these requirements come from. Law firms and their clients don’t create rules that limit themselves for no reason, and many of these requirements are more than a suggestion or matter of preference.
Requirements and constraints generally come from five different sources:
1. Legal and Regulatory Requirements
Legal and regulatory requirements are the most obvious source of constraints for law firms and legal departments. For example, the European Union’s General Data Protection Regulation (GDPR) or the UK’s Data Protection Act (DPA) may require that certain data be stored in a specific jurisdiction, or that certain practices be followed when processing personal data. For global companies or law firms that support global clients, requirements such as these often create compliance challenges that require careful planning and execution to manage effectively.
In addition to data protection laws, law firms and legal professionals within corporations may also be subject to professional “regulations” like those promulgated by the American Bar Association (ABA) and state bar associations in the US or the Solicitors Regulation Authority (SRA) in the UK. These regulations or ethical rules may impose additional requirements on how law firms and legal departments can procure and deploy AI solutions.
2. Risk Management Frameworks
Risk management frameworks are another important source of constraints for law firms and legal departments. In some cases, these frameworks may be incorporated into legal or regulatory requirements, such as when laws reference publications like those from the National Institute of Standards and Technology (NIST) or the International Organization for Standardization (ISO). In other cases, these frameworks may be adopted in response to industry practices or through contractual requirements, such as is often the case for SOC 2 or ISO 27001.
You can learn more about risk management frameworks and how they typically apply to legal technology solutions in our Risk Management for Legal AI article.
3. Insurance Requirements
Nearly all law firms and many corporate legal departments operate with external insurance coverage, and these insurance policies often impose additional requirements on how law firms and legal departments can use technology. Even in cases where organizations are self-insured or have captive insurance companies, these requirements are often still present in the form of internal policies.
For example, as cyber insurance becomes both more important and more expensive, insurance companies are increasingly requiring that their policyholders follow specific practices when handling sensitive data. Furthermore, issuers of Professional Liability/Indemnity insurance are now requiring transparency from their policyholders regarding the use of AI and other technologies that may impact the quality of legal services provided, as well as the risk of malpractice.
4. Client Preferences
Client preferences are another important source of constraints for law firms and legal departments. For example, a law firm client or corporate subsidiary may request that certain data be stored in a specific jurisdiction, or that certain practices be followed when handling their matters. Even when these preferences are not based in strict laws or rules, their internal procurement or risk management policies may require that law firms and legal service providers adhere to specific practices.
These client preferences are often documented in the engagement letters or outside counsel guidelines (OCGs) that law firms and legal departments use to govern their relationships. However, these preferences may also be communicated verbally or through other informal channels. Given the recent attention to AI technology, law firms should expect clients will be starting conversations if they haven’t already.
5. Internal Policies and Economics
Internal policies are another important source of constraints for law firms and legal departments. For example, a law firm or legal department may have internal policies that govern the use of certain technology or the handling of certain data. These policies sometimes align with the four types of requirements we described above, but they may also be based on other considerations, such as leadership’s own preferences or experiences.
In addition to these sources of constraints, law firms and legal departments must also consider the practical economic realities of their own operations. For example, a law firm or legal department may have a limited budget for technology or they may have limited technical expertise in-house. These practical realities may also constrain how law firms and legal departments can procure and deploy AI solutions.
Common Requirements
Regardless of where the requirements come from, law firms and legal departments should expect that they will need to comply with certain rules when procuring and deploying AI solutions. The process of identifying and managing these requirements is itself an ongoing challenge, but there are at least some common patterns and rules that law firms and legal departments can expect to encounter. Below, we’ll outline ten of the most common.
1. Rules related to data “at rest”
Rules related to data at rest are concerned with how and where data is stored. For example, a system may need to store certain data in a specific jurisdiction, or it may be required to store certain data using a specific type of encryption in an isolated way.
For legal AI solutions, these rules are no different. The contents of queries, documents, or responses sent to or from the AI solution are all data that should be handled in accordance with these rules.
2. Rules related to data in transit
Rules related to data in transit are concerned with how data is transmitted over a network. For example, a system may need to transmit certain data over a private network or VPN, or it may be required to transmit certain data using a specific type of encryption.
Just as with data at rest, the contents of queries, documents, or responses sent to or from the AI solution are all data that should be handled in accordance with these rules. In addition, since AI solutions often involve the use of multiple “layers” of APIs, it is important to trace the flow of data through all layers, even when they cross organizational boundaries.
3. Rules related to data processing
Rules related to data in use are concerned with how data is processed by an application. For example, a system may need to process certain data in a single-tenant environment or on a dedicated server, or it may be required to process certain data using an algorithm that can produce an “explanation” for its results.
Data processing is typically the most important aspect of legal AI solutions, and it is also the most complex. In addition to the data itself, the rules related to data processing may also apply to the algorithms used to process the data, the hardware used to run the algorithms, and the software used to run the algorithms. As in the case of data at rest and data in transit, it is critical to trace the flow of data through all layers, even when they cross organizational boundaries.
4. Rules related to data retention or deletion
Rules related to data retention or deletion are concerned with how data is kept or disposed of. For example, a system may need to retain certain data for a specific period of time, or it may be required to delete certain data upon request from a client or data subject.
Data retention and deletion are especially complicated topics for AI solutions, as they almost always involve cross-organizational data flows. For example, a corporate legal department may want to be able to delete data from its systems, but if its law firm, their legal technology vendor, or the third-party AI solution provider also has a copy of the data, it may not be possible to delete it from all systems. As AI providers increasingly covet the data they collect, it is extremely important to ensure that their data collection and model training practices align with your data retention and deletion requirements.
5. Rules related to business continuity or disaster recovery
Rules related to business continuity or disaster recovery are concerned with how data is backed up and how systems and their data are restored in the event of a disaster. For example, a vendor may be required to be able to restore data within a specific period of time.
Business continuity and disaster recovery are, once more, topics that often require understanding the “web” of parties who are involved in storing and processing your data. For example, if your legal technology provider relies on a third party like OpenAI or Google to provide AI services, you may need to understand how that third party handles business continuity and disaster recovery in addition to how your legal technology provider handles it. Conversely, just because OpenAI or Google can make certain representations about their capabilities like recovery point and recovery time objectives (RPO and RTO), that does not mean that these capabilities will implemented or available to you through your legal technology provider.
6. Rules related to authentication or authorization
Rules related to authentication and authorization are concerned with how users’ identities are verified and how their access to data and systems is controlled. For example, an organization may require multi-factor authentication for all users or minimum standards related to single-factor authentication.
As legal AI solutions may contain sensitive data, it is important to ensure that they are protected by strong authentication and authorization controls. In addition, it is important to ensure that these controls are implemented in a way that is consistent with your organization’s broader security requirements, such as through requiring the use of multi-factor authentication. As before, just because a cloud provider like AWS or Azure can make certain representations about their capabilities, that does not mean that these capabilities will implemented or available to you through your legal technology provider.
7. Rules related to audit or reporting
Rules related to audit and reporting are concerned with how data and systems are audited and reported on. For example, a system may need to provide audit logs to a client or regulator upon request, or it may be required to provide regular reports on the status of their systems and data.
Both insurance companies and regulators are increasingly interested in the use of AI in the legal industry, and as a result, it is important to ensure that your legal AI solution is capable of providing the audit logs and reports that may be required by your clients, insurance companies, or regulators. In addition, it is important to ensure that these capabilities are implemented in a way that is consistent with your organization’s broader operational security requirements and your data retention and deletion requirements.
8. Rules related to personnel
Rules related to personnel are concerned with how personnel are hired, trained, and managed. For example, an organization may be required to perform background checks on personnel or train them on specific topics prior to their engagement, or they may be required to employ personnel with specific types of experience, certifications, or citizenship.
As legal AI solutions often involve the use of third-party vendors and lower-cost resources to support the annotation of AI training data, it is important to ensure that these rules are applied through the entire supply chain. For example, if a law firm or legal department is required to perform background checks on personnel, this requirement should cascade down to any third-party vendors or contractors that the law firm or legal department engages to support the AI solution.
9. Rules related to third-party vendors
Rules related to third-party vendors are concerned with how third-party vendors are selected, managed, and monitored. For example, an organization may need to perform due diligence on third-party vendors prior to engaging them, or they may be required to monitor third-party vendors on an ongoing basis.
As with personnel, supply chain management for vendors is especially important for legal AI solutions given the common use of layered service providers and open source software. Organizations should consider whether certifications or attestations such as ISO 27001 or SOC 2 are required for their vendors and their supply chain, or whether additional audit rights or other contractual protections are required.
10. Rules related to insurance or risk management
Rules related to insurance are concerned with how insurance is procured and maintained. For example, an organization may be required to maintain a certain level of cyber insurance coverage, or they may be required to provide a named insured endorsement to a client or regulator.
Given the potential for significant liability associated with the use of AI in the legal industry, it is important to ensure that your legal AI solution provider is covered by appropriate insurance and that your contracting practices related to risk are consistent with your usage of the tools. For example, if your legal AI solution provider is not covered by cyber insurance, you may want to consider whether surety bonds or other forms of financial assurance are appropriate.
In addition to these common types of requirements, law firms and legal departments may also encounter other types of requirements based on their specific circumstances. For example, a law firm or legal department may be required to follow specific practices when handling extremely sensitive investigations or litigation.
Taking Action (in the “cloud”)
By understanding the sources and types of requirements that commonly occur in legal technology solutions, organizations can better understand how to evaluate and manage the risks associated with them. While these requirements may sometimes seem daunting, there are a number of common strategies that organizations can use to manage them.
In particular, while not all legal technology solutions are “Software-as-a-Service” (SaaS) or “cloud”-based, many of them are. In our Cloud for Legal AI article, we discuss four strategies that many organizations use to address these requirements when using cloud-based solutions.
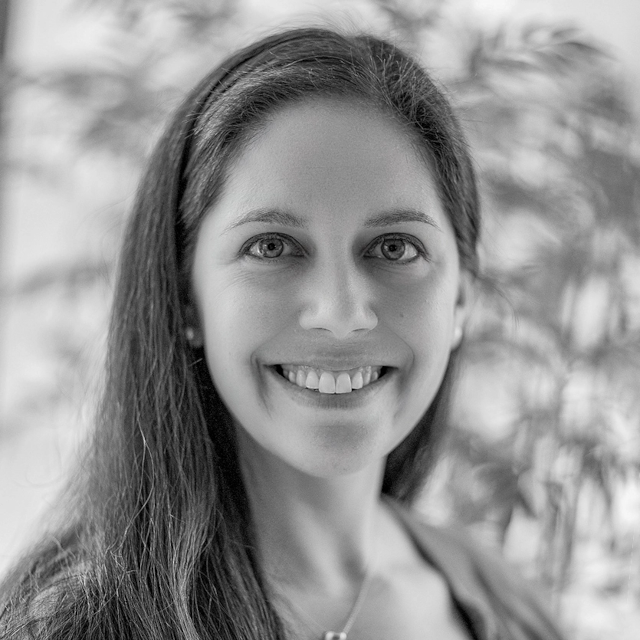
Jillian Bommarito, CPA, CIPP/US/E
Jillian is a Co-Founding Partner at 273 Ventures, where she helps ensure that Kelvin is developed and implemented in a way that is secure and compliant.
Jillian is a Certified Public Accountant and a Certified Information Privacy Professional with specializations in the United States and Europe. She has over 15 years of experience in the legal and accounting industries.
Would you like to learn more about risk management for AI-enabled legal tools? Send your questions to Jillian by email or LinkedIn.