· 5 min read
Kelvin Legal LLM Stands Out in FTC Landscape
FTC Tech Summit confirms importance of Kelvin Legal LLM
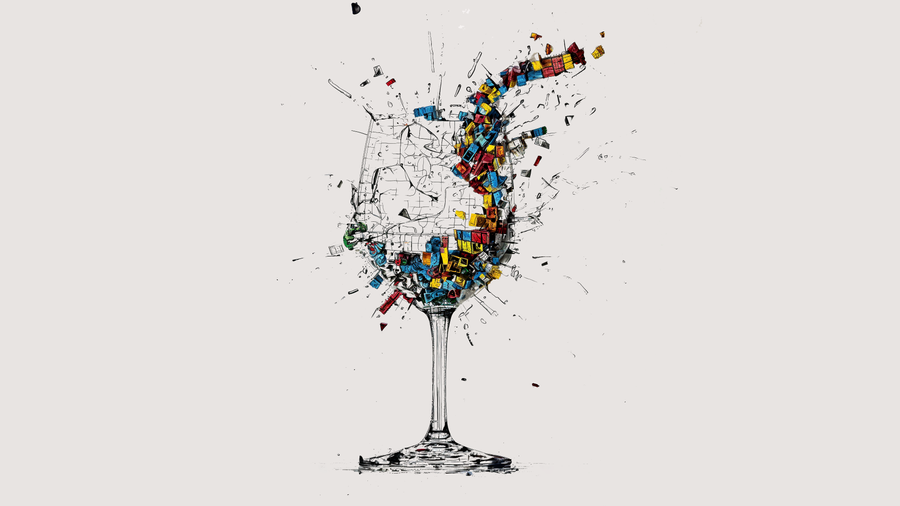
Rarely has there been an FTC event as eagerly anticipated as last week’s FTC Tech Summit. On paper, this mundane January 25th session brought together a “diverse set of perspectives across academia, industry, civil society organizations, and government agencies for a series of conversations on AI across the layers of the technology stack[.]” In reality, the event was possibly one of the most exciting and energetic yet, touching hot topics in tech competition and AI at once.
Throughout the day, one message came up over and over again: building or using AI does not absolve you of your contractual or statutory requirements.
Yes, AI is strategically important and potentially transformative for the better. Yes, it’s hard. But no, you cannot wantonly ignore laws and agreements just because it’s AI.
Using AI Legally
From a risk management perspective, the use of AI-powered tools or products has to be considered in light of a broad range of possible requirements, including how it fits into the overall AI strategy. While the discussion during the Tech Summit focused on requirements from regulatory sources, a prudent assessment of use cases and specific AI tools builds off of this to include requirements related to organizations’ own risk tolerances, use cases, and other internal and external factors.
While the majority of legal requirements sit squarely with the developers of models or products, organizations using these solutions are still responsible for ensuring that their use is legal; a panelist from the CFPB noted, if a company can’t comply with consumer obligations under financial laws based on their use of a certain AI product, they shouldn’t be using it. The procurement process, particularly within the legal industry, should include an assessment of how a product or tool helps or hinders an organization’s ability to comply with the law.
Building AI Legally
Multiple speakers during the Summit, including government officials, tech founders, and reporters, expressed concerns around the collection and usage of data for training AI (including large language models). One panelist suggested that one reason that companies are so secretive about their model training data is precisely because of the regulatory or judicial actions they might be subject to as a result, either immediately or in the future. Anyone who has ever interacted with a toddler who took a cookie off the counter when they weren’t supposed to has seen firsthand how quickly humans can learn to hide behavior that they know is wrong.
There are valid business reasons to keep training data private; no one would fault Coca Cola for not being transparent with their recipe, as this trade secret is their most valuable asset. On the other hand, the public has the general expectation that the recipe does not contain illegal ingredients. Interestingly, this analogy applies even when you consider that the recipe once contained ingredients that later became illegal - but the ingredient was not used when it was illegal. To apply this to models is more difficult, as once data is “baked into” a model’s weights, it can’t be removed; while research into this exact problem is being conducted, as of today, there’s no real solution.
While the entire IP ecosystem is in a quantum state with respect to models and copyrights - both of their training data and their weights - you’re not excused from the law just because you’re training a model.
FTC Chair Khan reiterated that model disgorgement is going to be a significant method of enforcement against companies that have engaged in anticompetitive, deceptive, and unfair business practices to train those models. The FTC has required companies to delete data and disgorge models because of unfair or deceptive practices related to the collection of data used to train those models, and in some cases they have even prohibited the companies from engaging in the building or use of those types of models for a period of time going forward.
Squeaky Clean LLMs
From a business continuity perspective, we want to ensure that our Kelvin Legal Data OS continues to work, regardless of the outcome of regulatory or legislative action, judicial decisions, or client preferences. Our decision to train a foundation model from scratch, with totally clean provenance highlights our commitment to our clients and our belief that effective and efficient LLMs can be built without stealing data.
Based on the commentary during the Tech Summit, as well as feedback that we’re hearing in the market, we’re feeling pretty good about the squeaky clean large language models that we’re building from scratch at 273 Ventures.
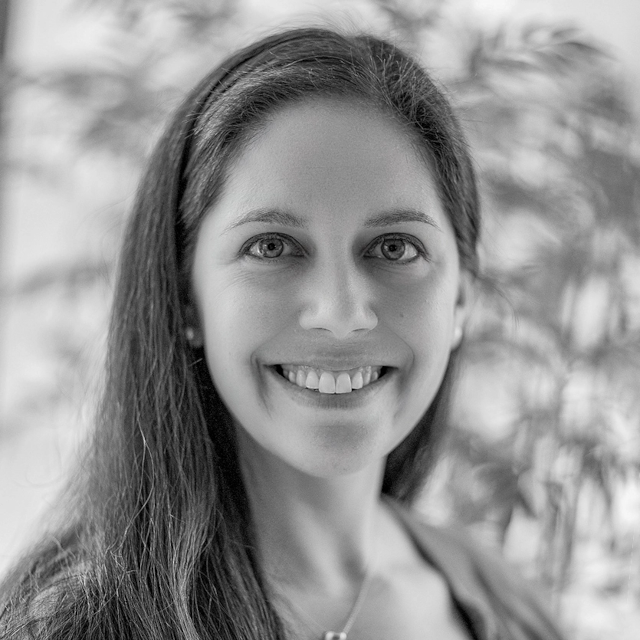
Jillian Bommarito, CPA, CIPP/US/E
Jillian is a Co-Founding Partner at 273 Ventures, where she helps ensure that Kelvin is developed and implemented in a way that is secure and compliant.
Jillian is a Certified Public Accountant and a Certified Information Privacy Professional with specializations in the United States and Europe. She has over 15 years of experience in the legal and accounting industries.
Would you like to learn more about risk management for AI-enabled legal tools? Send your questions to Jillian by email or LinkedIn.