· 5 min read
Why We Built the Kelvin Legal DataPack
Not just another Pile of Risk.
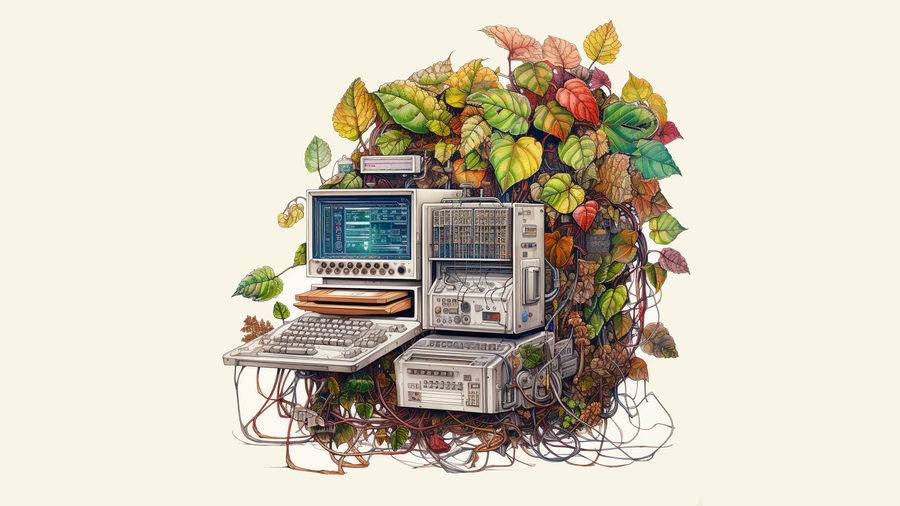
Soon after we started 273 Ventures, we began to research a topic clearly near and dear to our hearts: training data. Every real AI model starts with training data, and a great deal of subsequent performance is determined by the breadth and quality of this data. This is especially true in the legal domain, where the syntax and substance of language is critical.
We didn’t approach this problem lightly. In addition to Dan and Mike’s backgrounds as leading scholars in applied AI, I am a Certified Information Privacy Professional for the US and Europe and one of the first ForHumanity Certified Auditors for AI systems. Over the last three years, my professional focus has been on the ethics, security, and compliance of AI models and their training data.
So, the three of us set out to review available datasets for training. We read hundreds of publications available on large model construction, as well as any dataset-specific publications like The Pile. Our conclusion, unfortunately, was that there was no training dataset that checked the boxes. Two primary issues confronted every option we reviewed: either data was collected under breach of contract or there were issues with intellectual property rights.
Existing Datasets and Breach of Contract
Many of the most popular datasets used in large language models today include data that was collected under circumstances that possibly entailed breach of contract, particularly with respect to websites’ terms of use or service. Automated scraping or the use of other bots to retrieve content from websites often violates the sites’ terms, although responsible automated retrieval is sometimes explicitly allowed. This distinction is generally not made between the separate sources within a dataset, resulting in the entire dataset becoming tainted.
It’s important to note that frequently organizations use datasets in ways that do not violate those publisher’s terms of use or service; the issue at hand here is at least one degree removed from this, as it relates to whether a dataset publisher breached contracts during their development of that dataset. While organizations may not be directly exposed to legal risk related to this breach of contract, they are almost certainly exposed to the related business continuity risks. If organizations rely on these datasets (either directly through their own use or indirectly through their use of a LLM trained on them), legal actions, such as injunctions, could significantly impact their operations. For organizations that utilize at-risk datasets or models, it’s essential that they consider alternatives in the event of business interruption as part of their AI risk management process.
Existing Datasets and Intellectual Property Rights Issues
Within the public discourse and legal actions, copyright violations are the most well-known and discussed issue surrounding training data for LLMs. Recent complaints, including multiple class action lawsuits against companies who develop LLMs, center around the use of copyrighted materials in training data.
While some models and datasets provide complete transparency of the sources of their training data, many models are either closed or only provide open weights, leaving users in the dark when it comes to their exposure. Regardless of whether there are these types of unknown issues or there are known issues related to IP rights (due to the use of copyrighted material in datasets), both lead to business continuity risks for organizations relying on these datasets or the related models.
Developing a Solution
Like so many others, we were driven to develop our own solution when we couldn’t find one in the market. Enter: the Kelvin Legal DataPack. Our work was driven by a set of principles that would directly address our concerns with existing datasets; we had to have a clear understanding of ownership, intellectual property, and commercial reuse.
We only obtained data from sources where our retrieval did not violate the sources’ terms of use or service. In other words, we ensured that our retrieval and compilation of documents did not result in a breach of contract that would impact our dataset.
Furthermore, we only obtained data from sources with clear intellectual property rights that explicitly allowed reuse of data for commercial purposes. Our goal was to amass data that was not only free of use restrictions, but that was also high quality from the start. Rather than ingesting huge swaths of uncurated data and hoping to filter out the “garbage,” we opted to instead begin with high-quality data.
We focused on legal domain data that comes from high quality legal and financial sources, much of which was actually produced by attorneys and other legal professionals. We have collected practically all laws and rules from key jurisdictions, including the US, the UK, and the EU, with an end result of over 150 billion true tokens and more than 200 billion BPE tokens, as traditionally measured by GPT models. The Kelvin Legal DataPack covers numerous languages, including English, Spanish, German, and French.
We’re excited to be able to offer a dataset that has clean provenance and commercial licensing terms; we believe that this type of dataset will enable organizations to capture the power of large language models without the exposure to risks historically associated with them.
If you’re interested in learning more about the Kelvin Legal DataPack, you can read our announcement or email hello@273ventures.com for more info.
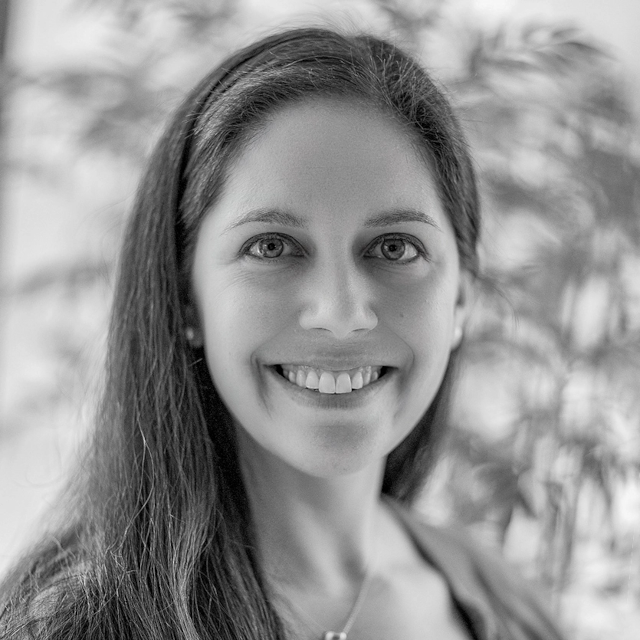
Jillian Bommarito, CPA, CIPP/US/E
Jillian is a Co-Founding Partner at 273 Ventures, where she helps ensure that Kelvin is developed and implemented in a way that is secure and compliant.
Jillian is a Certified Public Accountant and a Certified Information Privacy Professional with specializations in the United States and Europe. She has over 15 years of experience in the legal and accounting industries.
Would you like to learn more about risk management for AI-enabled legal tools? Send your questions to Jillian by email or LinkedIn.