· 6 min read
Why Data Matters - External Forces in AI
Like winds that impact a ship’s sails, external forces impact the data used to train, tune, and augment AI models.
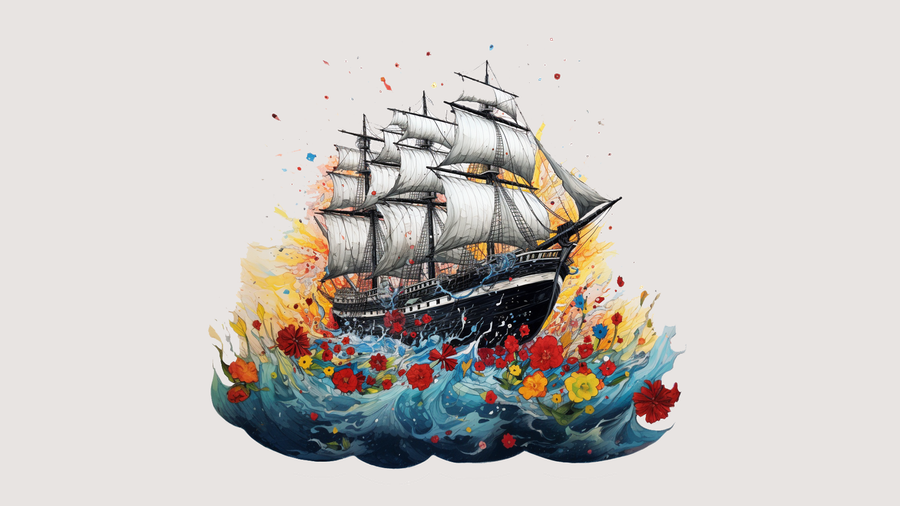
Just as sailboats cannot move forward without a sail, AI cannot function without data. Skilled sailors are adept at reading and responding to the wind to best capture it in their sails; skilled users of AI tools and models must be similarly capable of observing the external forces that impact the data that propels them forward. In our last post we discussed the need for large amounts of high quality data to support model training, tuning, and augmentation (the “sails” of the ship). Today we’ll turn our attention outside of the models themselves and instead focus on the external forces that drive the constraints and requirements of the data used for these purposes (the “wind”).
The source and contents of datasets often matters due to constraints or obligations imposed by third parties; most frequently this is through laws and regulations, judicial outcomes, or commercial contracts. The data sources or datasets used have a cascading impact on the downstream models and products or services powered by those models.
Data Protection Regulations
Data protection laws and regulations are typically the first hurdle that comes to mind when performing a risk assessment related to the data used to train, tune, or augment an LLM. While obligations vary by jurisdiction, they often include a right to be forgotten; these types of obligations introduce significant complexity with respect to how they can be addressed, particularly once a model has been trained on that data. While numerous organizations and governmental agencies across the world are researching ways for models to “unlearn” certain data, at this point there is not a clear, simple way for this to be done. The most effective approach is a proactive one, where the dataset is as “clean” and high-quality as possible. While it’s unlikely that any useful dataset is entirely free of personal information, within the legal industry there are many high quality sources of data that are, such as laws, rules, and regulations. The use of these types of sources, as opposed to crawls of websites, is much less likely to introduce data that is subject to data protection regulations.
AI Regulations
While most AI-specific regulations are in their infancy, with most jurisdictions currently negotiating proposed rules, they are still important to consider as part of a broader AI risk management framework and strategic AI planning. The proposed EU AI Act, for example, would have a significant impact on both the development and use of models with respect to the data on which they are trained or tuned; there are likely going to be positive obligations related to disclosure of copyrighted data used in training datasets.
As is frequently the case in the United States, regulation occurs more quickly at the state level than the federal level; while there is not yet proposed federal legislation that has significant traction, numerous states have introduced legislation to address the training and use of AI. Currently the federal agency that is most focused on the data used in training or tuning models is the Federal Trade Commission. To date, the most notable actions that the FTC has taken with respect to AI training data have been the imposition of algorithmic disgorgement for the implicated organizations. Improperly obtained training data led the FTC to require the organizations to delete both the tainted training data and the models that were trained on that data. The FTC has taken a clear stance that the source of data (and how it was obtained) matters.
Judicial Uncertainty
There have yet to be any decisions from the current cases surrounding copyright infringement in training data used for models, but multiple cases are in progress covering infringement in literary works, code, and art. The fact that these cases have not been decided yet means that both the developers and users of models have no clear guidance on how intellectual property rights, such as copyright, are going to be handled in this new AI-driven world. Some countries, such as Japan, have proactively addressed this matter, rather than waiting for it to be decided by the courts - Japan does not consider the use of copyrighted material in training data to be an infringement. In the US, however, the most recent relevant decision is still the 2015 Authors Guild decision regarding fair use of copyrighted material, which leaves substantial room for uncertainty with respect to how copyrights and fair use apply to training data.
To continue beating the risk management drum, the options for the creators and users of models (particularly LLMs) are to accept the risk - likely a “wait and see” approach based on the court’s decisions, mitigate the risk - perhaps by identifying a backup source of training data or model, or avoid the risk by utilizing training data (and models trained on said data) that don’t violate copyright.
Contractual Restrictions
One final constraint to discuss today: contractual restrictions surrounding models and the datasets on which they are trained. Even if a dataset is free of copyright violations and regulatory obligations (or if those obligations are met), the data may not be able to be used for training, tuning, or augmentation purposes if there are contractual restrictions or prohibitions. Sometimes the restrictions are included in the terms of use or service, other times they are covered by the dataset or model’s licensing terms (such as noncommercial Creative Commons licenses). In some instances, the constraints are included in contractual agreements like Master Services Agreements (MSAs), outside counsel contracts, or clickwrap agreements.
Restrictions on the use of data may be imposed by an aggregator and distributor of data, or directly from the data source (which could include customer-imposed restrictions). An understanding of these restrictions is essential to proper model usage; a model that is licensed for commercial use doesn’t make sense if the training data prohibits commercial use, yet these continue to proliferate in the AI marketplace. Regardless, it’s important to make sure that the underlying data is allowed to be used in the context of the ultimate end-state.
Why Data Matters to You
As part of developing an AI strategy, it’s imperative that organizations determine how they’ll be using datasets, models, or AI-enabled products and services. Due to legal requirements (both governmental and contractual) and the judicial uncertainty surrounding AI and training data, having a clear understanding of the relevant data and corresponding obligations is essential to surviving the external forces that impact the current technological landscape.
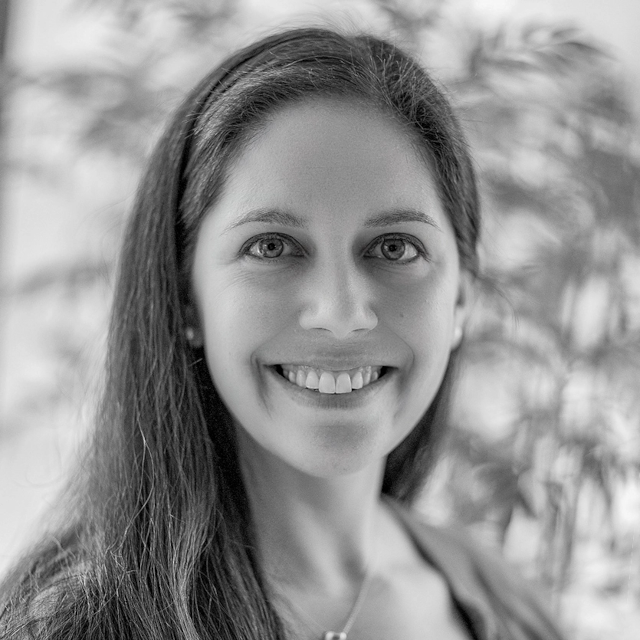
Jillian Bommarito, CPA, CIPP/US/E
Jillian is a Co-Founding Partner at 273 Ventures, where she helps ensure that Kelvin is developed and implemented in a way that is secure and compliant.
Jillian is a Certified Public Accountant and a Certified Information Privacy Professional with specializations in the United States and Europe. She has over 15 years of experience in the legal and accounting industries.
Would you like to learn more about risk management for AI-enabled legal tools? Send your questions to Jillian by email or LinkedIn.