· 8 min read
Removing Roadblocks in Revenue Recognition with AI
Route 606, as the crow flies.
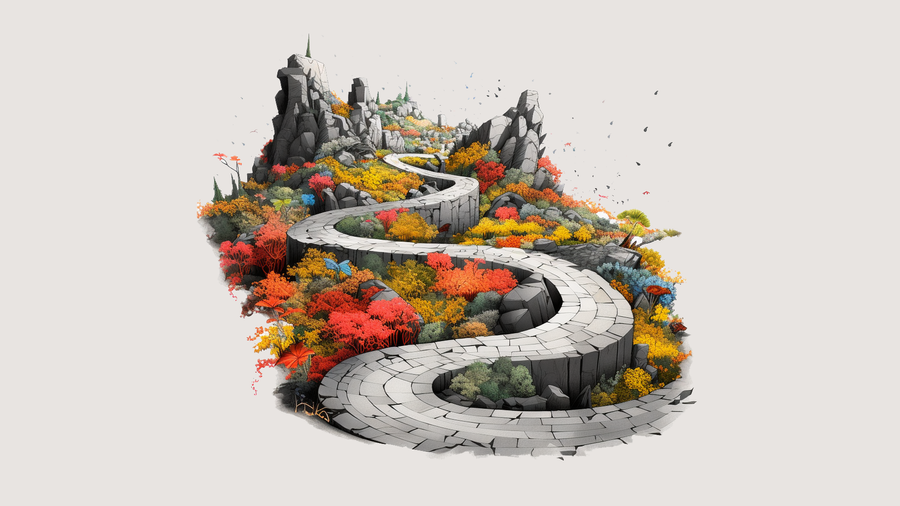
Like water down a mountain slope or the course of a road, we often take the path of least resistance in our daily work. Yet just like streams twist or switchbacks bend, our paths too often seem to be far from straight or simple. Revenue recognition, a task that nearly all large companies face, is full of such frustrating and roadblocked paths.
Thankfully, recent advances in large language models like GPT-4 have changed the landscape of legal and accounting technology. Many of the monotonous frictions that slowed ASC 606 or IFRS 15 determinations can now be addressed by extractive and generative AI, and forward-looking organizations now see a straight path to implementing streamlined revenue recognition programs.
To better understand what is now possible, it helps to understand what revenue recognition is, and why every legal, accounting, or financial stakeholder should care.
Cash vs. Accrual: When a Contract Dollar isn’t a Dollar
At first glance, you might think that a dollar of contract consideration is worth a dollar in a financial statement or accounting system. However, there are many instances where this is not the case, especially in the world of accrual accounting.
Unlike cash accounting, where revenue and expenses are recognized when real cash flows in or out, accrual accounting seeks to more accurately reflect the economic impact of transactions, regardless of when cash changes hands. Most large businesses, as well as all publicly traded companies, are required to use accrual accounting to communicate with their investors and stakeholders.
Integral to accrual accounting is the process of ‘revenue recognition.’ This is the point at which a specific transaction or event contributes to the earned revenue for a company. Revenue recognition under generally accepted accounting principles (GAAP) is driven by the criteria in ASC 606. There are five general steps that must be followed when determining when to recognize revenue:
- Identify the contract(s)
- Identify the related performance obligations within the contract
- Determine the transaction price
- Allocate the transaction price among previously identified performance obligations
- Recognize revenue as (or when) the performance obligations are satisfied
While there are certain types of contracts that are specifically excluded from this standard (such as leases and some financial instruments), the standard is intended to cover a broad range of transactions.
Identifying the Contracts
The terms and conditions directly impacting the revenue recognition process often lie hidden within contractual clauses, scattered correspondence, or data entries across diverse platforms, such as Master Service Agreements (MSAs), emails, Customer Relationship Management systems (CRMs), or buried deep within a set of exhibits and addenda. For companies with a large volume of such materials, the task of consistently and accurately identifying these critical terms impacting revenue recognition can be daunting.
Even when people know which documents include relevant information, finding the most recent version, confirming that the salesperson didn’t send a side-email with a concession to a customer, and identifying the specific clause is a sisyphean task once you consider that it needs to be done for every unique transaction.
This is where the power of AI-enabled tools steps in, significantly streamlining the process by allowing for systematic identification and analysis of such terms. Our Kelvin Legal Data OS connects to local network filesystems and services, email, and other cloud-based products and systems (Office 365 products, Google Drive, Dropbox, and more) to ensure that organizations don’t miss any documents that contain important information for revenue recognition.
Identifying the Performance Obligations
A performance obligation is a promise in a contract with a customer to transfer a good or service to the customer. These obligations may be satisfied over time (such as for an annual subscription to a SaaS product) or may be completed at a single point in time (such as the sale of merchandise to a customer at a retail store). A single contract may include multiple performance obligations, some of which may or may not be separately identifiable. To further complicate the matter, the performance obligations stated within one contract may be impacted by obligations of one or more other contracts.
For example, a company may license a customized software solution to a client under one contract and provide maintenance and support of that software to the client for two years after delivery of the software. The performance obligations of the support contract are directly impacted by the delivery of the software. It’s important to note that these performance obligations can be scattered across numerous contract addendums, invoices, or even email communications. The ability to identify and connect related performance obligations is essential to an efficient and effective revenue recognition process.
Determining the Transaction Price
In some cases, determining the transaction price is as straightforward as identifying the price of a product that is payable upon delivery. In most cases, however, the transaction price is impacted by at least one complicating factor, such as contingencies, performance bonuses, credits for downtime or missed SLAs, discounts or usage-based pricing, and noncash consideration.
Kelvin uses natural language processing to analyze documents, which allows organizations to find information without knowing how it might appear in the contract. For example, if a salesperson agreed to provide a rebate to a customer if their utilization drops below a certain percentage, you don’t have to know whether they offered a “discount,” a “rebate,” or a “credit” in order to find the relevant information.
Allocating the Transaction Price
The goal of this step is to allocate the portion of the transaction price that reflects the consideration that an organization expects to be entitled to in exchange for fulfilling a specific performance obligation; in other words, splitting the transaction price determined in the prior step between all of the performance obligations identified previously.
In reality, this is typically a challenging process, as many organizations may not have standalone prices for items or services that are typically bundled or that involve variable consideration that is attributable to multiple performance obligations.
This step demonstrates the importance of “human-in-the-loop” with respect to AI: while AI tools like Kelvin can substantially decrease the amount of time required to identify relevant clauses and make determinations about treatment, human review should still be included as a step in this process. Models can be trained on how an organization makes judgment calls (for example, by using historical and current market conditions, internal margin information, and desired profit objectives to develop an expected “cost-plus approach” for allocating the transaction price), but given the financial penalties associated with issuing materially inaccurate financial statements, CEOs and CFOs have a vested interest in ensuring that the output is accurate.
Recognizing Revenue
The final step in the process is actually recognizing the revenue, although this final “step” may really be multiple steps if performance obligations are satisfied over time. The standard dictates that performance obligations are satisfied when (or as) a customer obtains control of an asset. In this context, control is a customer’s ability to obtain substantially all of the benefits remaining from and direct the use of a service or good. There are numerous criteria that dictate whether revenue is recognized over time, including whether the organization’s performance creates an asset that it can use and whether it has an enforceable right to payment for that asset in its current state.
The use of a tool like Kelvin helps bring together all of the relevant elements that may be scattered throughout the organization’s systems to help determine to what extent (if any) revenue is recognized at a specific point in time. For example, an organization might have a master service agreement that outlines acceptance criteria for projects, a statement of work that specifies the work to be performed and milestones that will be billed, a separate statement of work to provide support under a monthly retainer model, an email from the customer confirming acceptance of a particular milestone, and internal communications that indicate that the final milestone has not yet been reached.
On the Glide Path
For organizations who harness tools like Kelvin, the glide path to streamlined revenue recognition is now clearer than ever before. Time-wasting roadblocks throughout the process, from identification of contracts to allocation among obligations, can be made to disappear in short order. Furthermore, systems that safely harness generative AI like Kelvin can also allow stakeholders to effectively summarize and “question” these contracts, simplifying the process of what-if analysis and stakeholder communication.
As LLMs continue to expand the horizon of legal and accounting technology, forward-looking organizations can directly impact their financial health and quality of life by implementing programs like this. By embracing approaches like those powered by Kelvin, businesses can get to where they’re going - an accurate, consistent characterization of their financial performance - with a simpler, faster route. Leave the long and winding route for your next ramble in the woods, not revenue recognition.
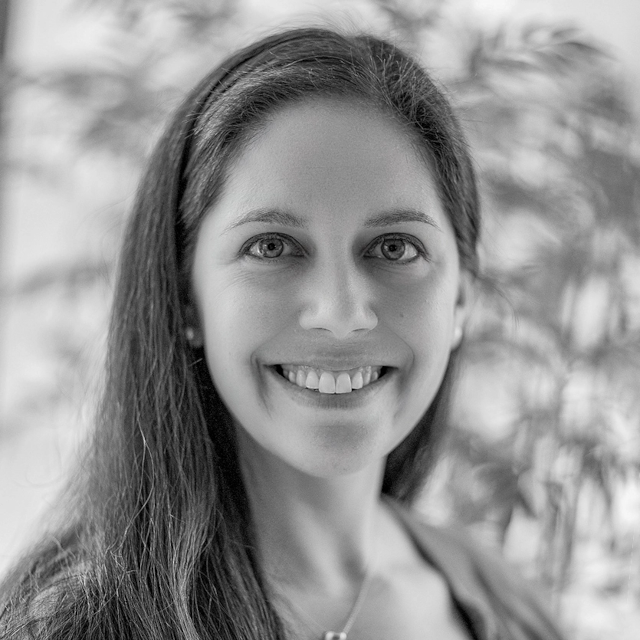
Jillian Bommarito, CPA, CIPP/US/E
Jillian is a Co-Founding Partner at 273 Ventures, where she helps ensure that Kelvin is developed and implemented in a way that is secure and compliant.
Jillian is a Certified Public Accountant and a Certified Information Privacy Professional with specializations in the United States and Europe. She has over 15 years of experience in the legal and accounting industries.
Would you like to learn more about risk management for AI-enabled legal tools? Send your questions to Jillian by email or LinkedIn.