· 10 min read
Future-Proofing Your Legal AI Strategy
Charting a course in choppy waters requires a clear idea of what lies over the horizon.
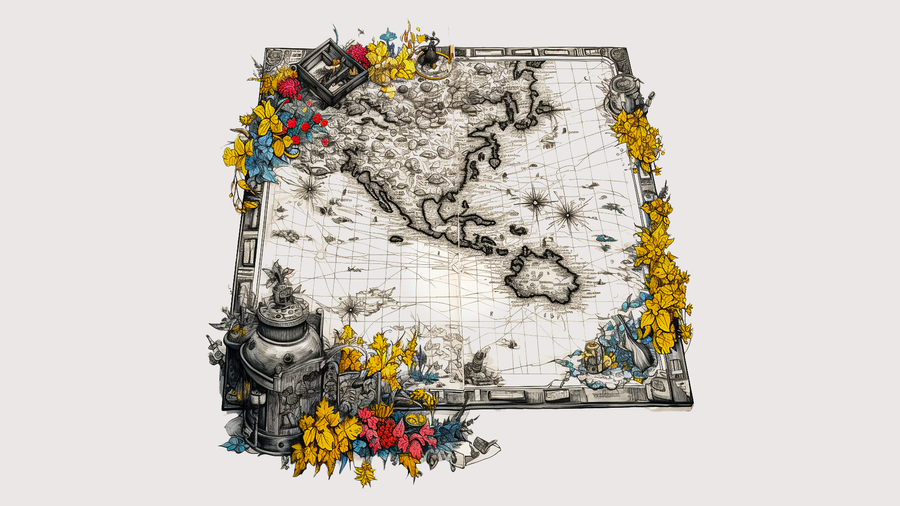
As the AI revolution surges forward like a tidal wave, it has never been more important - or difficult - for law firms and legal departments to look over the horizon.
But with a new academic or commercial announcement every day, how can decision-makers possibly chart a course in such choppy waters?
The winds of innovation may have changed direction by the time you read this article, but we still aim to equip you with the sextant of knowledge to help you “navigate the seas” - that is, to construct a framework for understanding how research and vendor announcements should influence your strategic planning.
To begin this voyage, we’ll embark by understanding how AI models are constructed and deployed.
The Lifecycle of an AI Model
The first step in understanding how to future-proof your AI strategy is to understand the lifecycle of an AI model. While the specifics of this lifecycle will vary from vendor to vendor, the general process follows a cycle that begins with data collection, continues through training and fine-tuning, and culminates in deployment and integration. Each of these stages is essential for the successful functioning of the model.
Data Collection
This is the first stage in the AI model cycle, as the data that’s collected will feed the model with the information needed for it to “learn.” The quality of the data used to train the model will have a significant impact on the model’s performance. The amount of data required will vary depending on the complexity of the model and the task it is being trained to perform. For example, a model that is being trained to draft any kind of legal document will require a much larger dataset than a model that is being trained to classify causes of action in a narrow area of law.
Until very recently, the overwhelming theory was that more data would result in better models. However, a number of existential questions related to copyright, privacy, and fair use surround the data collection process, though a discussion of these matters is beyond the scope of this post. To date, most law firms and legal departments have not explored to what extent their own private information could be used, but this is changing.
Training and Fine-Tuning
Once the data has been collected, the model developer next selects a type of model to train and fine-tune. The model developer will then label the data collected in the prior phase and train the model on it. The labeling and training process can be computationally expensive, so it is has historically required access to specialized hardware and labor. Given these historical costs and the limited availability of specialized hardware, most usage of AI like large language models has been through cloud-based services like OpenAI’s API.
The training process is iterative, and the model will typically be trained multiple times until it reaches a level of performance that is deemed acceptable. The training process is also where the model is fine-tuned on or aligned with a specific task. In some cases, AI model vendors like OpenAI may allow third-parties to fine-tune their models, but these services can often be impractical for cost and flexibility reasons due to vendor business models.
Deployment and Integration
Once the model has been trained and fine-tuned, it can be deployed to production. The deployment phase brings the model into active service, enabling it to deliver value in real-world situations. This involves making the model available to users so that they can interact with it.
The deployment phase is also where the model is integrated with other systems. This is a critical step in the process, as it is where the model is connected to the systems that will be used to interact with it. The integration process can be complex, and it is important to consider the security and performance of the model when integrating it with other systems. Platforms like our Kelvin Legal Data OS are designed to make this integration process as simple as possible, but thoughtful planning is still required given the complexity of law firm and legal department workflows and IT environments.
The Crucial Trio of Data, Software, and Hardware
At a high level, we can understand the lifecycle by focusing on three key types of assets: data, software, and hardware. Each of these assets is essential for the successful functioning of the model, and each is subject to its own economics and trends. Understanding each of these is essential for understanding how to future-proof your AI strategy.
Data
Data forms the lifeblood of AI models, providing them with the knowledge base they need to operate. Without suitable data, even the most sophisticated model is useless. However, there are challenges here. Most available AI models rely on public data, which may not fully represent the types of documents commonly encountered in legal work. Moreover, reliance on public data can also lead to compliance issues, which can be particularly significant in the legal industry.
Software
Software is the mechanism that defines the model architecture and states how it processes and interprets this data, effectively giving the AI model its “mind.” While software used to be a significant barrier to entry, this is no longer the case. The democratization of software, via open source platforms and affordable alternatives, has opened up AI to organizations of all sizes.
Hardware
Hardware is the engine behind the operation, executing the necessary calculations to turn the software instructions and data into trained models and responses to user inputs. Historically, this has primarily involved specialized Graphics Processing Units (GPUs), typically from Nvidia.
The Changing Winds
Recent years have seen significant changes in each of these areas, with the winds of change blowing in different directions. The past few months have seen even more tempestuous changes, as the release of ChatGPT and GPT-4 have only further accelerated the pace of investment and innovation.
Data
The data landscape is changing rapidly, as both the volume, variety, and regulation of data are all evolving. Many open source and academic projects have released their own datasets based on the work of companies like OpenAI and Google, and these datasets can now be used to train models.
Some organizations have also demonstrated the value of proprietary, domain-specific datasets. For example, Bloomberg has built their own transformer-based model on their own proprietary financial dataset, which has demonstrated superior performance in domain-specific tasks compared to more general models like OpenAI’s GPT.
However, the data landscape is also becoming more regulated, as governments and private parties around the world have increasingly sought to understand and regulate the use of both training data and the outputs from the models themselves. In some cases, regulators have even sought to temporarily halt the use of certain models, resulting in serious business continuity risks for companies relying on third-party models, as we noted in our previous post on risk management for legal AI.
Software
The software landscape is also changing rapidly, as the open source community has released a number of new tools and models that make it easier to train, deploy, and use models. While many of these tools are still in their infancy or are targeted at consumer use cases, they are rapidly evolving and demonstrate potential patterns of use for enterprise adoption in the legal industry.
In particular, academic research focused on running larger models on smaller hardware has resulted in a number of techniques that have dramatically increased the accessibility of AI models. For example, lower-resolution 4-bit or 8-bit models allow consumer-grade hardware to run models that were previously only available on specialized, enterprise hardware. This trend is likely to continue, as the economic incentives for increased affordability are significant in light of the current scarcity of enterprise GPU hardware.
Separately, new research from both commercial and academic groups has pioneered the use of alternative model architectures. While these models are still in their infancy and have not yet been proven to perform or scale as well as transformer-based models like GPT, there is increasing evidence that a new generation of model architectures may remove many current limitations related to memory requirements or context windows.
Hardware
The hardware landscape is also changing rapidly, as the demand for enterprise GPU hardware has dramatically outstripped supply. This has resulted in a number of new entrants into the market, as well as changing business strategy for both Nvidia and cloud vendors with existing GPU hardware. For example, Nvidia has recently announced new GPU “appliances” under their DGX/EGX/HGX lines that are bundled with software or models, allowing organizations to purchase an AI platform instead of relying on cloud vendors like Microsoft or Amazon.
Separately, hardware and cloud vendors like Intel and Google have also announced new offerings that could overturn Nvidia’s dominance in the market. For example, Google’s new TPUv4 hardware, which they are making more widely available to customers, is designed to run models like GPT-3 and GPT-4 at scale both more quickly and sustainably than Nvidia’s GPUs.
Likewise, Intel has recently announced that its Xeon Scalable processors can be used to train and execute large language models without relying on any other specialized hardware. Such a development could dramatically increase the accessibility of AI models, as it would allow organizations to run models on their existing hardware without needing to purchase specialized servers or scarce GPUs. Such a development could also remove current limitations like small context windows, as the memory available to CPU-based models is significantly more scalable than GPU-based models.
Over the Horizon
Given these trends, it’s clear that the world of AI is set to undergo significant changes, dramatically expanding what is possible for most law firms and legal departments. As we sail into this exciting future, it’s essential to future-proof your AI strategy.
The best way to do this is to ensure that your AI strategy is flexible and adaptable, allowing you to take advantage of new developments as they emerge. For example, if you are looking at point solutions or SaaS offerings, ensure that you have the ability to export your data or limit your subscription to a shorter term than you might otherwise. This will allow you to switch to a new solution if a better one emerges.
Organizations with the desire to truly control their future can also consider investing in their own AI platform like our Kelvin Legal Data OS. Platforms like Kelvin allow organizations to have the best of both worlds so that they can rapidly leverage AI while also retaining the ability to adapt to new developments as they emerge.
As with any voyage, the key to success is to be prepared to adapt to the changing conditions you will inevitably encounter. By focusing on flexible, scalable solutions and avoiding long-term lock-in, organizations can ensure that they are well-positioned to take advantage of the opportunities that AI offers. Given the pace of change in the AI today, this is the best way to ensure that your organization is ready for the bright future ahead.
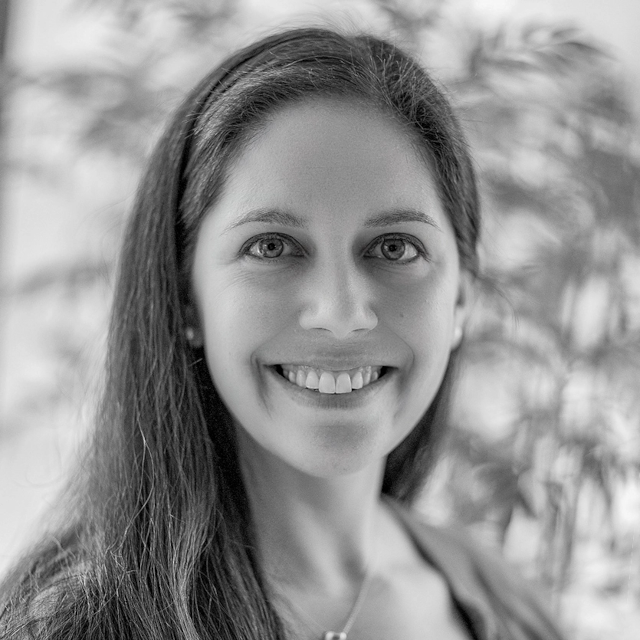
Jillian Bommarito, CPA, CIPP/US/E
Jillian is a Co-Founding Partner at 273 Ventures, where she helps ensure that Kelvin is developed and implemented in a way that is secure and compliant.
Jillian is a Certified Public Accountant and a Certified Information Privacy Professional with specializations in the United States and Europe. She has over 15 years of experience in the legal and accounting industries.
Would you like to learn more about risk management for AI-enabled legal tools? Send your questions to Jillian by email or LinkedIn.