Β· 5 min read
From the Foothills to the Mountain Top - Two Arcs of Development for Generative AI & Law
We are prepared for the climb ahead
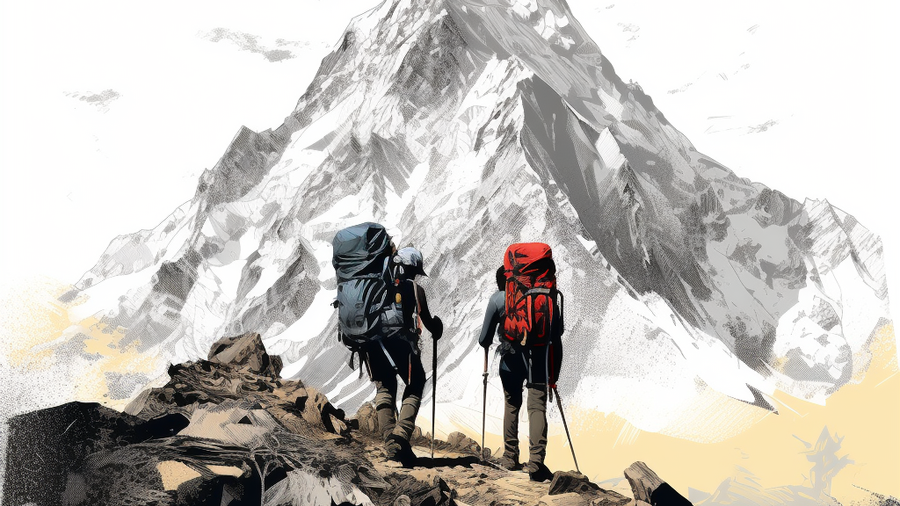
GPT-4 Passes the Bar in Zero-Shot but We Still are Only in the Foothills
It has been quite a year so far in AI & Law. The year began with the release of βGPT Takes the Bar Examβ in which 273 Ventures co-founders Michael Bommarito & Daniel Marin Katz evaluated GPT-3.5 on the multiple choice portion of the Multistate Bar Exam (MBE). This paper ultimately put them on the path to work on βGPT-4 Passes the Bar Examβ which was part of the GPT-4 launch back in March. As was noted in the paper, the bar exam results (including both the multiple choice and the essays) were really βlower boundβ results focused upon the raw capabilities of the leading Large Language Model (LLM).
Obviously, the Bar Exam results are pretty amazing in their own right but the results in many ways really understate the true nature of the potential of LLMs. The Bar Exam analysis is what is called a zero-shot analysis because it does not involve one of many potential engineering layers that might be built on top of these core capabilities. It is for these reasons that I am convinced that we are only in the foothills at this point β we have not even come close to reaching the mountain top. The graphic below offers a sketch of two arcs of development for Gen AI & Law β from the raw zero-shot capabilities of LLMs to much stronger results through combinations of data, tools, engineering and agents.
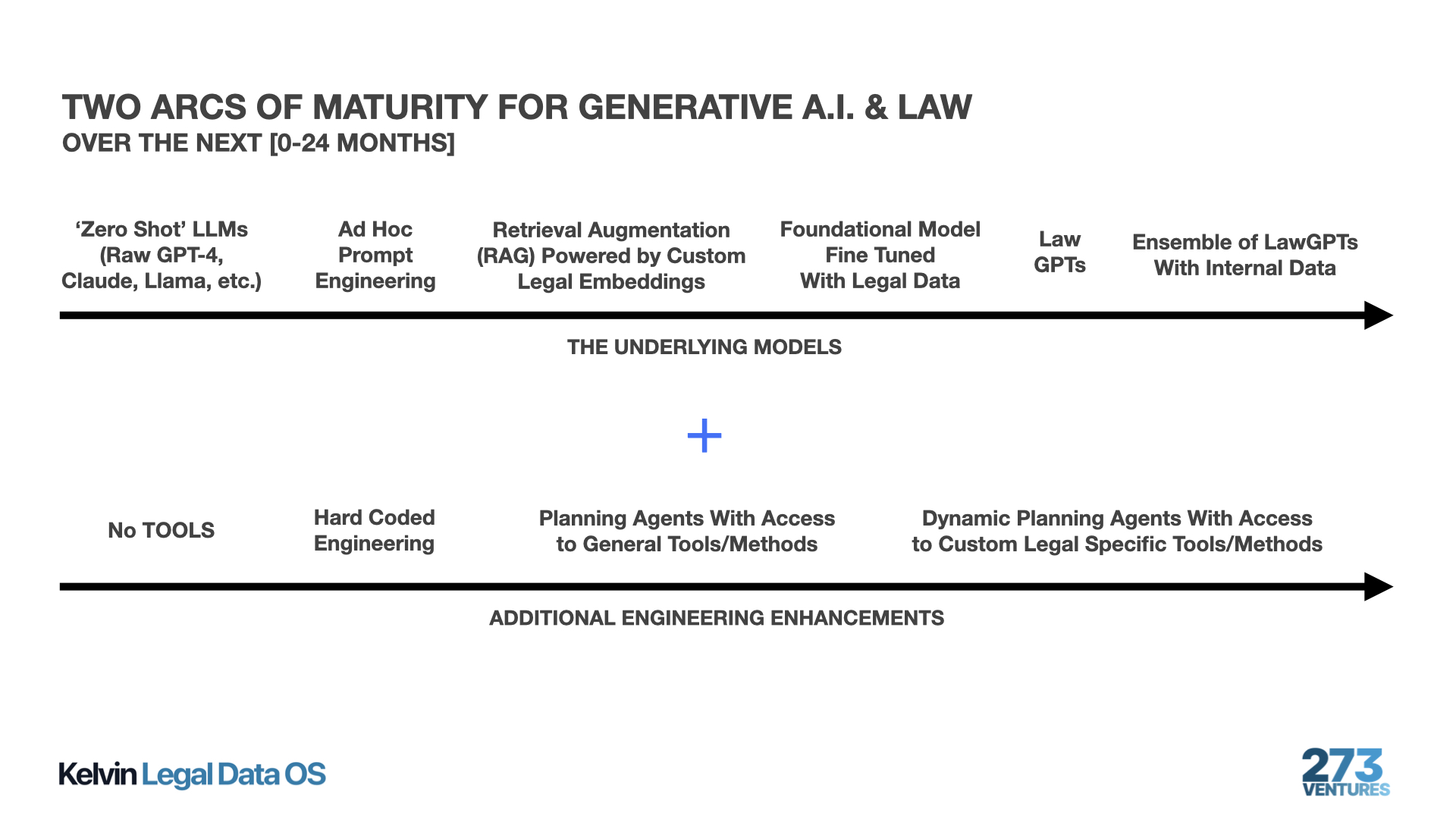
Ad Hoc Prompt Engineering is Not Really Engineering
Prompt Engineering has been all the rage this year. Donβt get me wrong. It can be useful and can help generate superior results when compared to the cold turkey results one can otherwise derive from an LLM. However, much of the ad hoc Prompt Engineering is not really engineering at all (as there is not really anything systematic being put into play). Namely, mapping between inputs and outputs in LLMs is difficult to truly systematize. The underlying cocktail of gradient descent and other techniques operating on giant inscrutable matrices makes it difficult to develop principled prompt-centric approaches that yield consistent high fidelity output.
RAGs2Riches and Fine Tuning a Foundational Model
One way to partially systematize the process of generating results is to use Retrieval Augmented Generation (RAG) powered by custom embeddings. We have highlighted some of the strengths and limitations of RAG as well as the utility of legal specific embeddings for retrieval augmentation. Indeed, we have 31+ Flavors of Kelvin Embeddings which we currently ship to customers or embed within our own offerings. These Kelvin Embeddings are built from the Kelvin Legal Data Pack which features 200B Tokens and Counting.
The Kelvin Legal Data Pack (or components thereof) are also currently being used to fine tune foundational models to support specific use cases. Model fine-tuning is one of the many forms of transfer learning in which one starts with an already pre-trained foundational model (GPT, Llama, etc.) and then re-trains it for another task. Various techniques to undertake this fine tuning exist such as through the use of LoRA, QLoRA or through the use of RLHF. Bottom line is that it is substantially less expensive and complex than building a foundational model from first principles. However, fine tuning ultimately faces an inherent ceiling on its potential performance. This ceiling can arguably be raised by a domain specific foundational model (e.g., a LawGPT).
LawGPTs and Planning Agents Using Custom Tools Developed for Use with Legal Data
Agents are now very much front and center in the discussion of Gen AI given their focus at the recent Open AI Dev Day. Ultimately, we see a world of legal specific foundational models (i.e., Law GPTs) which are connected to and combined with domain specific legal tools/methods through an orchestration layer (via legal agents). Our recently announced Kelvin Agent can orchestrate the implementation of the more than 50+ tools inside the Kelvin Legal Data OS. Namely, Kelvin OS features a series legal specific OCR (Kelvin OCR), spell checker (Kelvin Speller), tokenizer and sentence segmentation/chunker (Kelvin Document Index). It can also leverage the Kelvin Conversion Engine, Kelvin NLP, Kelvin Graph and many other tools. For a given problem, our Kelvin Agent will suggest potential plans for using the tools contained within the broad OS (and execute such plans at the behest of the user). Users can also design and execute their own plans, checklists or custom workflows using our no-code, low-code interface.
Two Arcs of Maturity for Generative AI & Law
We believe that the graphic above offers a useful summary of some of the trends that will come to define the next [0-24 months] in AI & Law. We are working to build that future here through our Kelvin Legal Data OS and associated Kelvin offerings. We are prepared for the climb ahead!
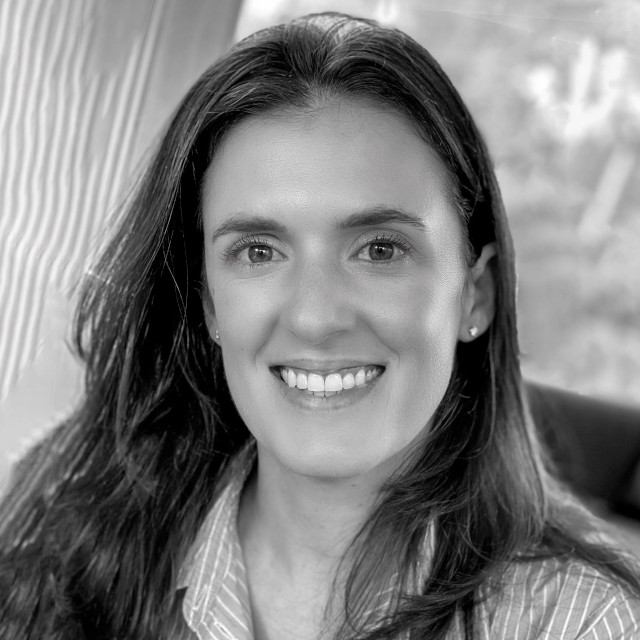
Jessica Mefford Katz, PhD
Jessica is a Co-Founding Partner and a Vice President at 273 Ventures.
Jessica holds a Ph.D. in Analytic Philosophy and applies the formal logic and rigorous frameworks of the field to technology and data science. She is passionate about assisting teams and individuals in leveraging data and technology to more accurately inform decision-making.
Would you like to learn more about the AI-enabled future of legal work? Send your questions to Jessica by email or LinkedIn.